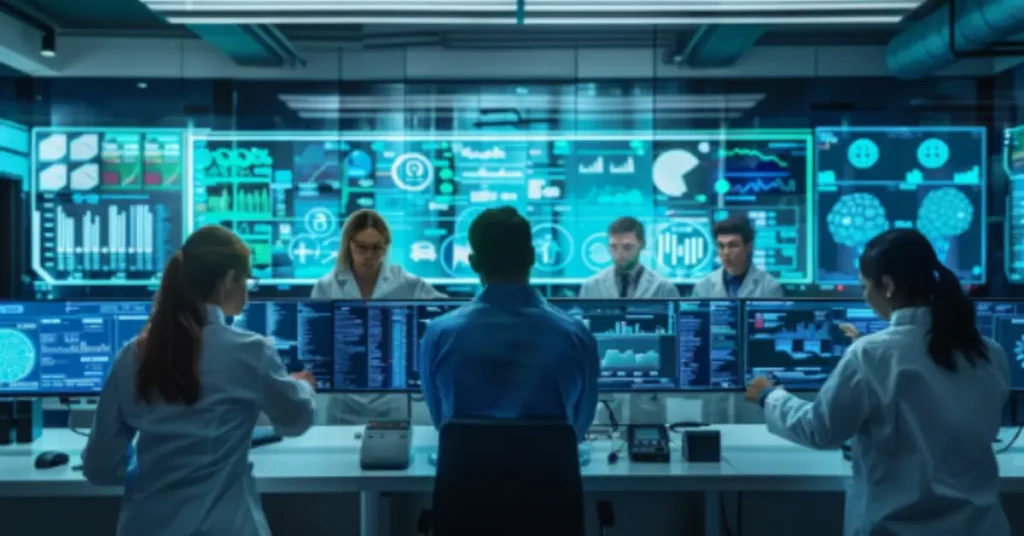
In this article:
Clinical trial performance metrics are essential for ensuring the success of clinical studies. These key performance indicators (KPIs) confirm that the data collected is accurate, complete, and aligned with regulatory standards. Without the right metrics, errors can slip through, timelines can stretch, and compliance risks may jeopardize the entire trial.
Imagine investing years into a clinical research project, only to face delays due to poor quality and integrity of data. Missed milestones, protocol deviations, and incomplete records can derail a study, costing millions and postponing access to life-changing treatments.
When the right metrics are in place, however, issues are identified early, workflows streamline, and regulatory approvals become more achievable.
What Is Clinical Data Management?
Clinical data management (CDM) is the process of collecting, cleaning, and managing data collected during clinical research to ensure its accuracy and reliability. Regulatory agencies like the FDA and EMA require high-quality data to evaluate the safety and efficacy of new treatments, making CDM a critical part of the clinical trial process.
However, managing data alone isn’t enough. To improve outcomes and align with business goals, research teams must also monitor clinical trial performance metrics. These indicators help identify areas where the process can be improved, enabling faster decisions and more reliable results.
Understanding Clinical Data Management Metrics
So, what exactly are metrics in clinical data management? They are tools used to measure how well data capture and management processes are performing. Metrics help teams evaluate whether trials are progressing on schedule, staying within budget, and aligning with protocols and regulatory expectations.
These metrics provide insight into operational performance, highlighting what’s working and where adjustments are needed. To be effective, they must be clearly defined, relevant, and grouped by purpose, such as time, cost, quality, or compliance.
Metrics typically fall into two categories:
- Leading indicators help detect potential problems early and guide real-time improvements.
- Lagging indicators assess past performance and support future planning.
While it may be tempting to track everything, the most valuable metrics are those that align with goals and highlight data points that provide insight. Metrics aren’t the end goal—they are tools for evaluation, collaboration, and continuous improvement throughout the trial life cycle.
The Importance of Metrics in Clinical Trials
Clinical data management metrics play a vital role in ensuring trials run smoothly, stay compliant, and produce reliable results. By tracking key indicators, research teams can spot problems early, improve workflows, and meet regulatory expectations.
1. Detecting Data Discrepancies Early: Metrics like query rate, error rate, and SDV help identify missing or inconsistent data. Catching issues early improves accuracy and prevents delays.
2. Improving Operational Efficiency: Tracking cycle times for this metric, such as data entry turnaround or query resolution, highlight workflow slowdowns. These insights help teams act quickly, avoid situations where the process is delayed, and maintain overall efficiency.
3. Ensuring Regulatory Compliance: Compliance metrics help ensure that clinical trial data meets regulatory standards set by agencies like the FDA, EMA, and ICH GCP. They support data integrity and audit readiness by:
- Tracking protocol deviations and audit findings
- Ensuring data is collected and processed according to Good Clinical Practice (GCP) guidelines
- Identifying and correcting issues before regulatory submission
- Reducing delays caused by incomplete or inaccurate data
- Minimizing the risk of compliance violations or rejected submissions
1. Data Quality Metrics
- Data Quality: Measures the consistency, accuracy, and completeness of information gathered during clinical research. Strong quality practices help establish confidence in study outcomes.
- Data Accuracy: Assesses whether the recorded data truly reflects the observed or reported events. Higher accuracy leads to more trustworthy analyses and strengthens the validity of the trial.
- Error Rate: Indicates the proportion of incorrect or missing data entries within the dataset. A lower error rate contributes to smoother data cleaning and improved study efficiency.
- Query Rate: Refers to the frequency of data issues or discrepancies identified during validation, typically measured per form or data field. A high query rate may reveal problems in data entry practices or protocol compliance.
- Data Completeness Rate: Measures how many required fields are fully filled out across all forms. Gaps may point to a weakness in oversight or inconsistent data workflows.
2. Data Processing and Timeliness Metrics
- Data Entry Timeliness: Measures how quickly data is entered into the system after patient visits—ideally within 24 to 48 hours. Delays in entry can affect timelines and site measure performance.
- Data Entry Turnaround Time: Captures the average duration between data collection and system entry. A shorter turnaround time supports efficient trial progress and better data visibility.
- CRF (Case Report Form) Completion Rate:Tracks the percentage of forms submitted accurately and on time. This metric is often used to assess experience across trial sites.
- Query Resolution Time: Measures the time taken to address and resolve queries raised during data validation. Quick resolution maintains data flow, supports database lock timelines, and enhances regulatory readiness.
- Time to First Data Review: Measures how soon the initial review happens post-entry. This helps teams maintain momentum and ensure agreement on early data quality.
3. Compliance and Regulatory Metrics
- Protocol Deviation Rate: Measures how often procedures stray from the approved protocol. Frequent deviations may affect the safety and efficacy of the trial and raise regulatory concerns.
- Audit Findings and Compliance Score: Reflects both the volume and severity of issues identified during audits. These findings help teams assess whether processes meet expectations and support the definition of improvement areas.
4. Patient and Site Performance Metrics
- Patient Recruitment and Retention: Measures the number of participants successfully enrolled and retained through trial completion. Low recruitment or retention can lead to underpowered studies and extended timelines.
- Site Performance Metrics: Monitors how well each location performs in areas like enrollment speed and data accuracy. It can also uncover variability between sites, which may affect consistency across a multi-site trial.
5. Data Review and Lock Metrics
- Database Lock Time: Tracks how long it takes to finalize the database after the last patient visit, preventing further changes. A timely lock ensures the trial stays on schedule for analysis and regulatory submission.
- Source Data Verification (SDV) Rate: Measures the percentage of trial data that is checked against original source records. Risk-based SDV strategies now optimize resources by focusing on high-impact areas while preserving data integrity.
6. Safety and Reporting Metrics
- Adverse Event Reporting: Measures how promptly and accurately adverse events are reported during the trial. Timely reporting supports patient safety monitoring and ensures regulatory compliance.
- Data Monitoring: Involves continuous oversight of collected data to detect errors, deviations, or trends. Effective monitoring—through automation and manual review—helps maintain protocol adherence and study quality.
7. Data Integrity and Security Metrics
- Data Access Logs and Security Incidents: Tracks who accesses or modifies clinical data and when these actions occur. Regular log monitoring ensures accountability, detects breaches, and supports data protection efforts.
- Data Encryption and Backup Compliance: Ensures clinical data is securely encrypted and regularly backed up to prevent loss or unauthorized access. Compliance with regulations like HIPAA and GDPR protects data integrity and patient privacy.
- Data Archiving: Refers to securely storing trial data post-study for regulatory reviews or future analysis. Proper archiving ensures accessibility, traceability, and long-term compliance with record retention policies.
By tracking a complete set of metrics across quality, timeliness, compliance, performance, safety, and security, clinical data management teams can better manage risk, ensure data integrity, and keep trials on schedule. Regular review of these KPIs leads to more informed decisions and continuous process improvement.
How to Improve Clinical Data Management Metrics
Tracking clinical data management metrics is essential, but the real value comes from improving them.
Below are actionable strategies to enhance efficiency and reduce common errors.
1. Implement Real-Time Monitoring and Automation
Manual data tracking is inefficient and increases the risk of errors, leading to delays in clinical trials.
Real-time monitoring and automation tools help by instantly detecting discrepancies, sending alerts for missing data or errors, and improving completeness through automated checks. These technologies reduce query rates and ensure timely data entry, enhancing overall study reliability.
2. Use Data Validation Tools to Improve Accuracy
Data validation is a clinical data management process that detects real-time errors, inconsistencies, and missing information. Data validation tools enhance accuracy by automating range checks, flagging impossible lab values, and identifying duplicate or inconsistent entries.
Using double data entry helps catch mistakes and keep data clean. AI tools can check for issues in real-time, making it easier to lock the database and submit accurate data to regulators.
3. Enhance Training for Site Staff to Minimize Errors and Queries
Well-trained site staff are essential for maintaining high data quality and minimizing queries in clinical trials. Training should focus on accurate data entry, avoiding common protocol mistakes, and handling queries properly. Ongoing education helps staff stay current with regulations and data management standards.
4. Improve Communication Between Stakeholders to Reduce Query Resolution Time
Delays in query resolution can hinder database lock and slow trial progression. Improving communication between sponsors, Contract Research Organizations, and site staff helps shorten response times, reduce bottlenecks, and ensure alignment on regulatory requirements.
Implementing a centralized communication platform allows stakeholders to track, discuss, and resolve queries in real time, ultimately enhancing data quality and accelerating the trial timeline.
Regulatory Standards and Industry Benchmarks in Clinical Data Management
Clinical data management must follow strict regulatory standards to ensure accuracy, integrity, and compliance. Regulatory agencies set benchmarks for key metrics to confirm that trial data is reliable for drug approvals. Understanding these standards helps sponsors and contract research organizations (CROs) maintain high-quality data and remain competitive.
Regulatory bodies establish detailed guidelines for collecting, managing, and reporting clinical data. Key regulations include:
- ICH Good Clinical Practice (GCP): Defines global standards for clinical data quality and integrity.
- FDA (U.S. Food and Drug Administration): Requires trials to follow data integrity principles such as ALCOA+—Attributable, Legible, Contemporaneous, Original, Accurate, and Complete.
- EMA (European Medicines Agency): Sets strict requirements for data monitoring, validation, and protocol adherence in EU-based trials.
These regulations directly influence key performance indicators like data entry timeliness, query resolution time, and protocol compliance rates, all of which contribute to accurate and efficient clinical trial operations. Meeting these standards not only supports data integrity and regulatory compliance but also reduces the risk of delays, rejected submissions, or trial termination.
In addition to regulatory requirements, industry benchmarks help sponsors and CROs evaluate performance and maintain competitive standards. Common benchmarks include:
- Data entry timelines: 24–48 hours after a patient visit
- Query resolution time: 5–10 business days for critical queries
- Error rate: Less than 1%
- Protocol compliance rate: 95% or higher
- Database lock timeline: 4–6 weeks post-last patient visit
Meeting or exceeding these benchmarks helps ensure smooth trial execution, improves data quality, and increases the likelihood of timely regulatory approval.
Conclusion: The Value of Clinical Data Metrics
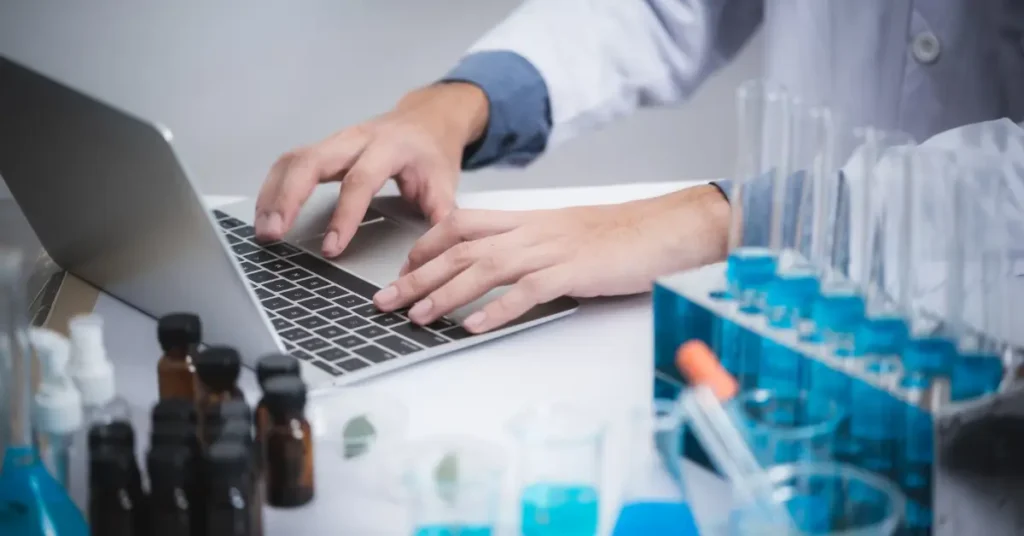
Clinical data management metrics play a critical role in the success of clinical trials. By tracking indicators across data quality, processing, compliance, site performance, safety, and security, research teams can identify issues early, improve operational efficiency, and maintain regulatory readiness.
Well-defined and actionable metrics support better decision-making, reduce trial delays, lower operational costs, and ensure data is complete, accurate, and audit-ready. Whether it’s monitoring query resolution time, protocol deviations, or database lock timelines, each metric contributes to building trust in the results and securing regulatory approval.
At the heart of this process is having the right tools. Our clinical data management platform is designed to help teams track, analyze, and improve key trial metrics,supporting cleaner data, faster timelines, and smoother compliance from start to finish.