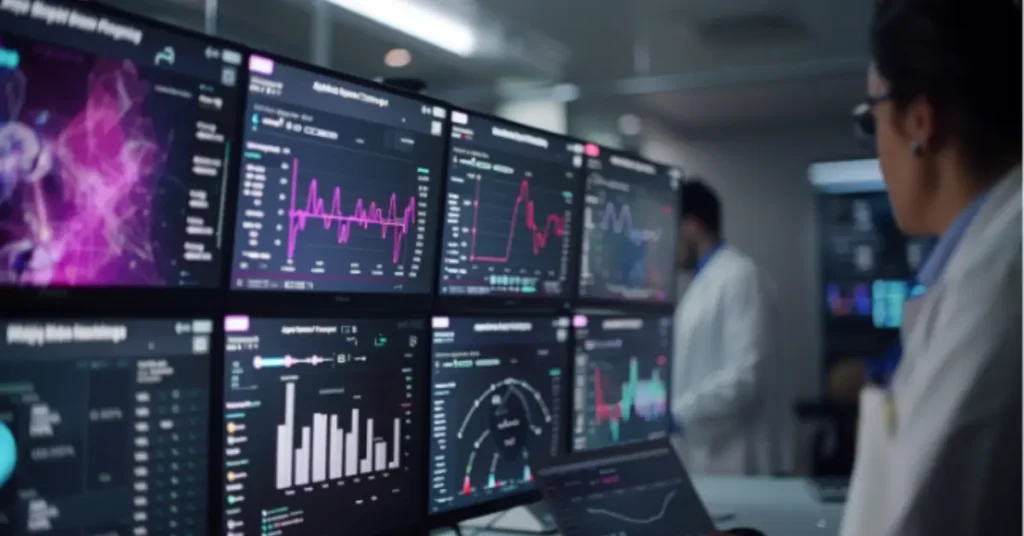
In this article:
Decentralized clinical trials (DCTs) have experienced significant growth in recent years, particularly following the global pandemic’s shift toward remote healthcare operations and digital-first solutions. This shift sparked a wave of digital transformation in how clinical studies are designed and managed.
Technologies such as wearables, mobile apps, and electronic data capture (EDC) systems have enabled the collection of vast amounts of clinical trial data outside traditional research sites. As a result, real-time data processing from multiple locations and sources has become both a possibility and a challenge.
While these innovations have expanded access and accelerated timelines, they also introduce new layers of complexity. Research teams must now navigate issues related to accuracy, data consistency, system integration, and regulatory compliance.
This article examines the most pressing clinical data management challenges in decentralized trials and offers insights into how they can be addressed with the right tools and strategies.
5 Common Clinical Data Management Issues in Decentralized Trials
DCTs offer numerous benefits, but they also introduce complexity, particularly in data management. As clinical research becomes more digital and remote, challenges in clinical data management are becoming harder to solve. Research teams must focus on navigating challenges like data accuracy, integration, and compliance.
Below are the top issues they face when handling data in DCTs.
1. Handling Large Volumes of Data from Multiple Sources
In decentralized clinical trials, data isn’t limited to site visits or hospital labs. It continuously streams from wearable devices, mobile apps, and in-home monitoring tools, 24/7, creating a constant flow of information..
This continuous stream generates massive datasets. A single wearable might track heart rate, sleep, and step count every minute. Multiply that by hundreds of participants, and you get millions of data points.
The challenge is not just storing this data, but also filtering what matters. Not every point is valuable. Researchers need more innovative tools to cut through the noise and highlight what’s relevant.
In traditional clinical trials, data were limited to scheduled visits, making it easier to manage. However, with DCTs, the volume and speed require advanced tools, like AI3, for real-time analysis and early health insights. These tools help analyze data efficiently and support faster decision-making.
2. Ensuring Data Privacy and Cybersecurity
Remote data collection introduces serious data security risks1. In decentralized clinical trials, sensitive patient information travels across various networks, cloud platforms, and devices, each step potentially introducing additional vulnerability.
Cyberattacks in healthcare are increasing. Hackers often target clinical trial databases to steal personal health data or intellectual property. In some cases, they demand a ransom to prevent data leaks, delaying research and damaging trust.
With numerous data sources and endpoints involved in DCTs, the risk of breaches or unauthorized access increases. Without proper safeguards, protecting data privacy becomes a major concern across the trial lifecycle.
3. Maintaining Data Integrity and Quality Across Devices
Data integrity means information is accurate, complete, and reliable from collection to analysis.
In decentralized clinical trials, this presents a challenge because data is collected from a variety of devices and data sources. One participant might use a smartwatch, while another reports symptoms through an app. Without on-site investigators, errors or inconsistencies can go unnoticed.
Poor data quality can seriously impact a study. Inaccurate readings or incorrect inputs can distort findings and lead to false conclusions about a treatment’s safety or effectiveness.
When multiple tools and methods are involved, ensuring consistent and trustworthy clinical trial data becomes harder, making quality oversight a critical concern.
4. Standardizing Data Collection Across Devices and Locations
A major key challenge in decentralized clinical trials is the lack of standardization. Participants use different devices and live in varying environments, which affects how clinical trial data is collected and measured.
For example:
- One fitness tracker may calculate heart rate differently from another.
- Step counts or sleep quality can vary between device brands.
Environmental conditions, such as heat, humidity, or altitude, can also influence the results. A participant’s physical response may differ based on their location, even if they follow the same routine.
These inconsistencies make it harder to compare results and draw meaningful conclusions, highlighting the need for better alignment in how data sources are used across trials.
5. Meeting Complex and Evolving Regulatory Requirements
Clinical trials, especially global ones, must follow a wide range of regulations, and these rules often differ by country and change over time.
For example, data protection laws like GDPR in Europe and CPRA in California set strict guidelines on how patient data is collected, stored, and shared. These inconsistencies across regions can confuse international teams. What’s permitted in one place may violate laws in another.
In decentralized clinical trials, more data is gathered than ever before, leading to more reports of side effects or irregularities. This increase in volume adds to the pressure of managing timelines and reporting formats for pharmacovigilance.
Without systems that keep up with evolving rules, staying compliant becomes a significant burden, raising the risk of penalties, trial delays, and loss of trust.
How DCTs Increase Clinical Data Challenges
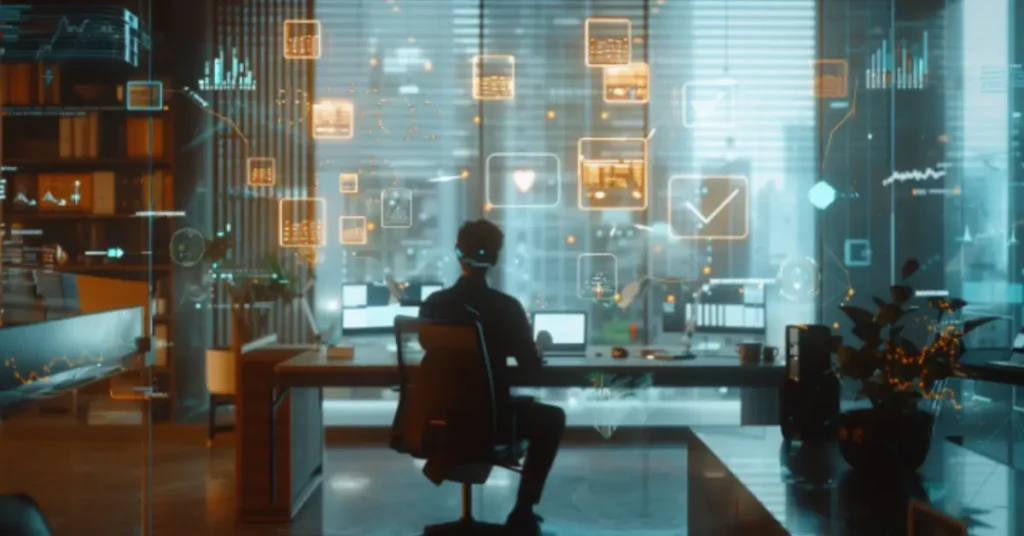
The shift from traditional trials to DCTs has changed how clinical data is collected, managed, and monitored. While DCTs offer greater flexibility and broader patient access, they also introduce new data management challenges that are often harder to resolve. One of the key issues is reduced oversight.
Less Oversight from Trained Professionals
In traditional clinical trials, trained staff collect data onsite, ensuring that procedures are followed correctly. Investigators can observe how measurements are taken, ask clarifying questions, and verify data accuracy, helping maintain high data quality from the start.
In DCTs, patients often use mobile apps, wearable devices, or home tools to report information independently. This removes professional oversight. If a patient misuses a device or misunderstands instructions, errors may go unnoticed.
Without in-person support, it becomes harder to catch issues early, making real-time monitoring and data validation tools essential to maintaining clinical data integrity in remote settings.
Managing Regulations in Global Trial Operations
DCTs make it easier to enroll patients from around the world. However, with that benefit comes the challenge of following different regulations in different countries.
For example, Europe’s GDPR and U.S. laws, such as HIPAA2 and CPRA, all set rules on data protection and patient consent. These rules often don’t align, which creates confusion during clinical operations. What’s allowed in one region might violate privacy laws in another.
Reporting adverse events, such as side effects, also varies. One country may require immediate updates, while another allows more time. Managing these differences slows down processes and increases compliance risks.
Traditional clinical trials usually operate under one regulatory body, which simplifies oversight. But DCTs must navigate overlapping legal frameworks, increasing the risk of delays, penalties, or shutdowns. These are real challenges in clinical data management for global teams working across borders.
Older Systems Weren’t Built for This Complexity
Many systems used in clinical research were built before DCTs became common. They were designed for smaller studies with limited devices and data collected at a single site.
Today’s clinical data flows from diverse sources, demanding rapid processing, thorough review, and robust security measures. Teams must also adapt swiftly to evolving regulatory requirements across multiple regions.
Older systems often lack the necessary tools for real-time data processing, centralized access, and compliance tracking. Without modern clinical data management systems, teams end up relying on manual workarounds that slow down progress and increase the risk of errors.
Solutions to Common Clinical Data Challenges
As DCTs grow, so does the need for more innovative ways to manage data. Outdated systems can’t address challenges like data volume, privacy, consistency, and compliance. Fortunately, modern clinical data management tools offer solutions that help research teams keep up. Here are two effective approaches:
Adopting a Unified Platform for Data Integration
One key solution is using a centralized system that connects all data sources, from apps to wearables, into a single platform. This helps reduce fragmentation and streamline data management processes.
A unified platform allows teams to:
- Collect real-time data from multiple devices and tools
- Organize information into consistent data formats
- Reduce errors from mismatched systems
- Monitor participant progress regardless of location or device
- Spot trends and outliers early
- Save time by reducing manual work
This approach supports more accurate analysis and minimizes the risk of mistakes caused by inconsistent data handling.
Using Tools That Prioritize Real-Time Monitoring and Compliance
DCTs require systems that allow teams to act quickly when issues arise. Tools that offer real-time data tracking and built-in compliance features are essential.
These tools help:
- Detect unusual patterns or missing data through automated alerts
- Improve data quality by catching problems early
- Ensure regulatory compliance by tracking consent and reporting
- Keep research teams informed of updates in global rules
By combining monitoring and compliance in one solution, teams can stay focused on research instead of struggling with paperwork and legal complexities.
Benefits of a Clinical Data Management Platform
Managing clinical data in DCTs can be overwhelming. Information comes from many data sources, in different data formats, and with varying levels of accuracy. A strong clinical data management platform simplifies this by unifying processes in one system.
1. Simplifies Collection, Tracking, and Analysis
A platform pulls data from wearables, apps, and home devices, then organizes it into a consistent system. This helps teams:
- View and analyze real-time data
- Spot trends faster
- Avoid missing or duplicate entries
- Compare results across participants
2. Improves Accuracy, Efficiency, and Compliance
With built-in checks and standard formats, platforms help:
- Catch errors early and improve data accuracy
- Automate tasks like data entry and reporting
- Stay aligned with evolving compliance rules across regions
3. Supports Remote and Multi-Site Coordination
These platforms make it easier to:
- Monitor participants remotely
- Coordinate across multiple trial locations
- Respond quickly to issues without delays
The result? Successful clinical data management, with fewer errors, smoother operations, and better data integrity.
What to Look for in a Clinical Data Management System
Choosing the right clinical data management system is key to running efficient and compliant decentralized clinical trials. Here are the essential features to consider:
- Scalability: Supports growing data volume, users, and devices, ideal for multi-site or global trials.
- Data Security and Privacy: Includes encryption, access controls, and aligns with data protection laws like GDPR and HIPAA.
- User-Friendly Interface: Simple navigation, custom dashboards, and real-time alerts for better visibility and faster action.
- AI-Based Analytics: Uses machine learning to detect trends, flag issues, and support data analytics and decision-making.
- Regulatory Flexibility: Adapts to evolving compliance needs across different countries, reducing legal risks and delays.
A well-rounded platform ensures successful clinical data management through improved speed, accuracy, and compliance.
Conclusion
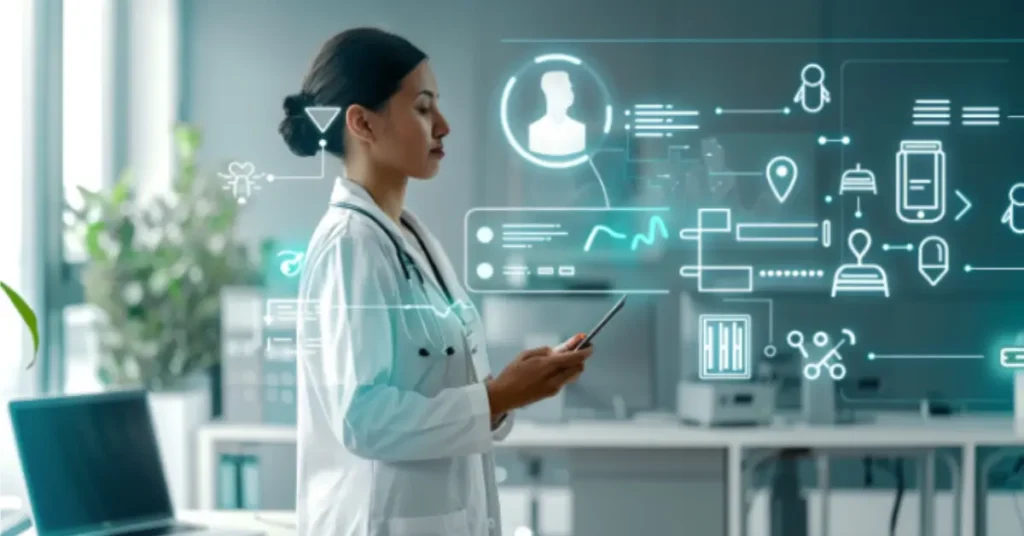
DCTs have changed the way research is done, but they’ve also made clinical data management more complex. With data coming from multiple devices, collected in real time, and spread across different locations, research teams face new challenges every day.
Some of the most significant issues include handling large volumes of data, protecting privacy, maintaining data integrity, standardizing data from different sources, and keeping up with changing regulations. These challenges can slow down trials, increase risks, and affect the quality of study results.
To keep pace with the demands of modern clinical research, teams need a solution that’s built for this environment. A secure, integrated clinical data management platform can help simplify workflows, improve data accuracy, and support compliance, no matter where the trial takes place.
To simplify your decentralized clinical trial workflows and overcome today’s data management challenges, explore CDConnect™, our secure and streamlined clinical data management solution.
Frequently Asked Questions
What Makes Clinical Data Management More Difficult in Decentralized Trials?
In decentralized trials, data comes from many sources, like wearables, mobile apps, and remote locations. This increases complexity, especially in terms of standardization, real-time monitoring, and maintaining data integrity. Without centralized oversight, managing consistency and accuracy becomes much more challenging.
How Can Research Teams Manage Data Overload in DCTs?
Data overload can be managed by using innovative filtering tools, real-time analytics, and centralized platforms that sort and prioritize relevant data. Automation and artificial intelligence help identify key trends while eliminating noise. These solutions make it easier to act on insights without being overwhelmed by raw data volume.
What Are the Biggest Risks to Data Quality in Remote Trials?
Common risks include inconsistent device outputs, user error, and lack of in-person oversight. When participants enter data themselves or rely on varying devices, accuracy may suffer. Without proper validation tools, even small errors can impact clinical outcomes.
How Can Sponsors Reduce Errors When Data Comes From Multiple Devices?
Sponsors can minimize errors by adopting platforms that support device integration, data standardization, and automated validation. Using consistent data formats across sources ensures better comparison and interpretation. Real-time alerts also help catch anomalies early in the process.
Sources:
- https://cloudian.com/guides/data-security/data-security-risks-policies-best-practices/#:~:text=Data%20security%20is%20the%20process,accidental%20damage%2C%20and%20human%20error.
- https://www.hhs.gov/hipaa/for-professionals/privacy/laws-regulations/index.html#:~:text=The%20Health%20Insurance%20Portability%20and%20Accountability%20Act%20of%201996%20 (HIPAA,was%20published%20December%2028%2C%202000.
- https://www.fda.gov/drugs/news-events-human-drugs/role-artificial-intelligence-clinical-trial-design-and-research-dr-elzarrad#:~:text=And%20its%20use%20is%20really,including%20in%20decentralized%20clinical%20trials.